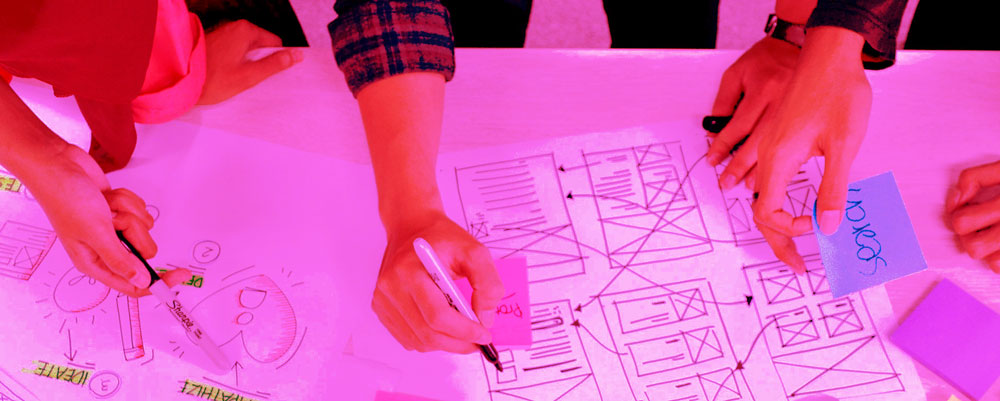
Some new key technologies are becoming more and more important in the retail business, and companies investing in this area are getting to the next level.
Driven by the computer vision momentum, machine learning, and artificial intelligence (AI), omnichannel platforms are rapidly evolving and impacting retail business, creating both a valuable opportunity to enhance customer experience and an important crossroad: proactively joining this transformation or establishing a defensive strategy to minimize the damage in the short-term.
Consumer retail shouldn’t disregard the possibility to adapt, transform and evolve, and many leading seller players encourage the transition of corporate processes to a more seamless client experience. As a matter of fact, the implementation of new technologies inside physical stores is rapidly interconnecting the offline and online worlds.
Retail technologies play a key role in this transformation, putting together the large puzzle of multiple retail processes through the omnichannel.
The following article introduces the role of computer vision applied to machine learning in the contest of the new omnichannel retail landscape, giving also an overview of the different technologies involved.
How to enhance client experience through computer vision?
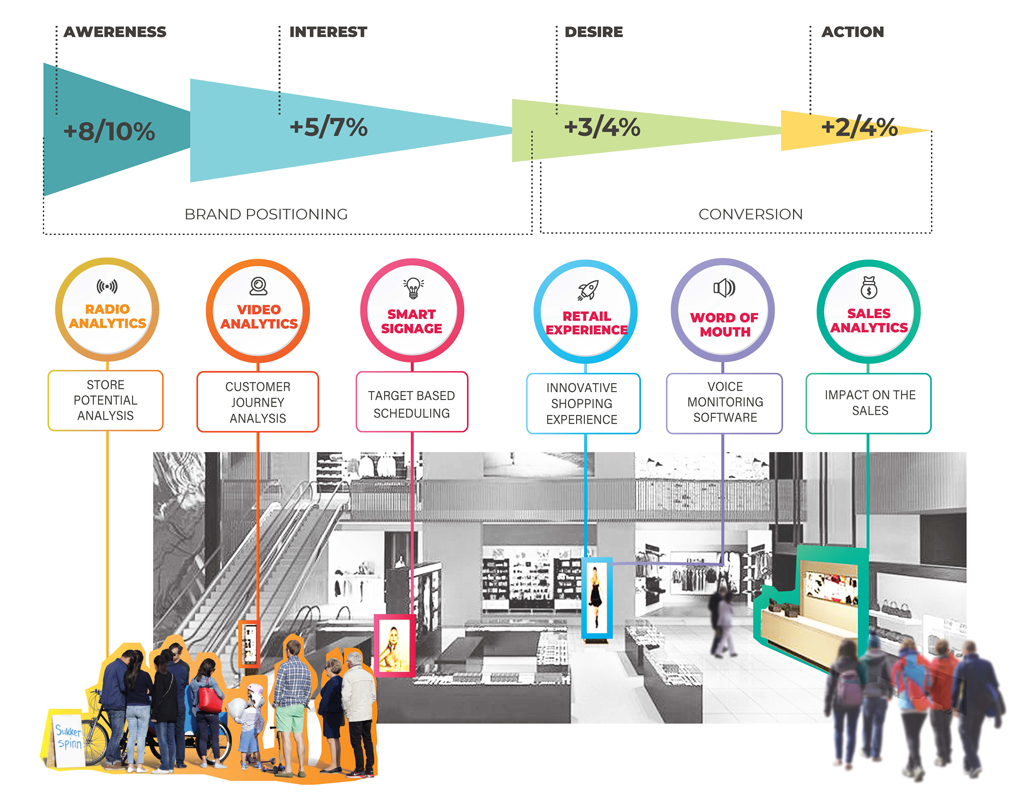
The data is the key.
As for the majority of human activities, the success rate is affected by the quality of the data, so taking decisions should be based on facts and verified patterns.
Our data must satisfy the below attributes:
Significance: data able to represent business relevant scenarios. Except in the specific cases, we need to focus our attention on most relevant KPIs and get a clear overview of potential customers (set aside low value data like the color of subject’s eyes):
- Clicks: what are the flows around my location, what’s my location potential?
- Sessions: how many of these potential subjects are accessing my store?
- Bounce Rate: how many of my in-store subjects interact with the experience?
- Duration: for how long these subjects stay inside my store?
Once we get confident with the mentioned plain KPIs, more elaborated data, as the integration with the cash register, can be processed and included.
Substantial: small data slip is expected and not material, my flow analysis won’t be negatively impacted by small counting errors (e.g., 99 subjects passed by but I revealed only 97, or 64 women passed by but I recognized only 62). Our major concern should be the consistency of my data during a certain period of time, consistency that will help me to identify relevant trends in my flows.
Broad: data has to be like puzzle pieces, and the final picture must make sense to my business. The final result is something significant that can help me to take informed relevant decision for my business. It becomes impossible to obtain the mentioned ideal qualitative data, if the system is not able to recognize the number of unique users (for example as per common people counting devices) or the time spent by a subject inside my store or area of interest.
Reliable: often taken for granted, the sensor mechanism must be able to perform in all weather conditions and On Premise in order to minimize TCO; ROI device can work offline, understand and solve possible new issues.
Updated: broader the info receipt and processing, lower will be the reaction capability. Notwithstanding the majority of strategic assessments can be elaborated after weeks or months from the data production, tactical actions require data analysis within few hours.
Data constitutes the puzzle pieces of the customer’s journey map, if these pieces are neither relevant, nor large, nor valuable, it won’t be helpful to put them together since I won’t be able to base my decisions on biased data.
In the next article we will kick off the data analysis method, identifying hands-on KPIs able to produce relevant information for the decision-making process inside-store.